A smiling woman unloads a dishwasher. The image, now embedded in a tweet, has been sourced from a collection of stock photos including other smiling women stationed at the same dishwasher. Partitioned into a neat, four-by-four grid, the image is topped with a familiar blue header and an apparently innocuous directive: “Select all squares containing a dishwasher.” Eight have been selected, as indicated by a familiar blue checkmark, but they contain the smiling woman, not the appliance. Is this a sophisticated critique of technology’s role in the gendered division of labor, or is it merely the handiwork of a misogynistic troll?
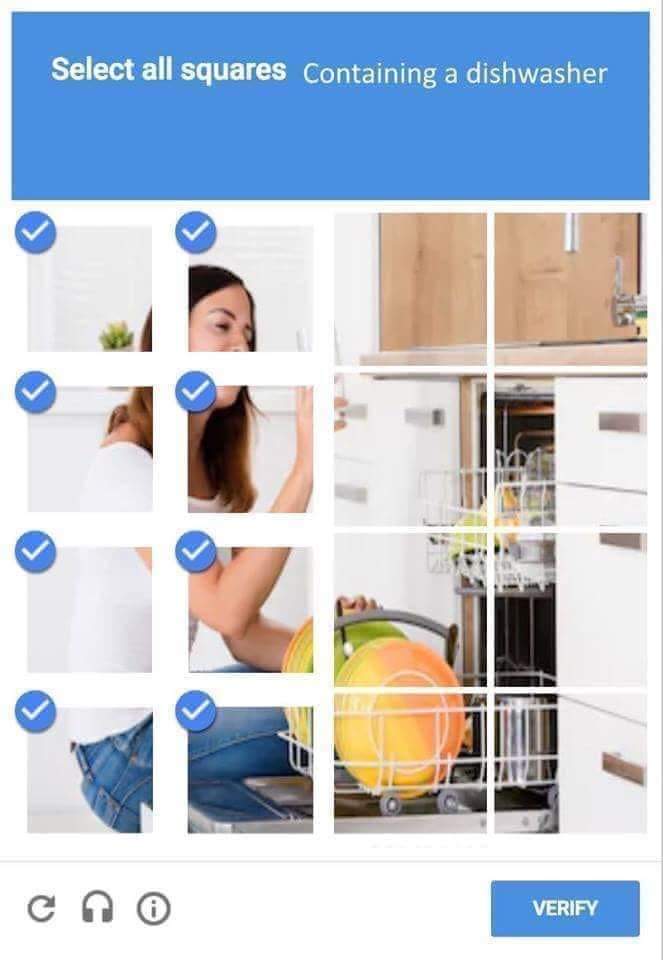
That this meme repurposes a CAPTCHA template is apropos. CAPTCHAs have been deployed for more than two decades as an ostensibly cut-and-dried way to distinguish between the sincere and the subversive — between humans and bots. Memes appear, on first glance, to serve the opposite function, muddling intentions and invoking indeterminacy for comic effect. Memes, however, also sharpen the distinctions that organize in-group membership, according to who “gets it” and who is offended or confused. Plumbing the depths of this interpretive gray zone has produced the uncanny brilliance of Weird Twitter, but it has also allowed systematic campaigns of harassment to reliably dodge content moderation policies on social media platforms. In both cases, attempting to articulate any definitive or intended meaning has the effect of stripping the communicative action of its context — @dril loses its wit, the alt-right gains an alibi. The line between irony and harm, in practice, is an inkblot.
Differentiating between humans and bots may appear as a straightforward technical operation, but the evolution of CAPTCHA suggests otherwise
This convergence between CAPTCHAs and memes suggests that the history of one can shed light on the problems presented by the other. Looking at both how and why CAPTCHAs have evolved since their inception in the late 1990s can reframe the contemporary concern over bots, misinformation, and the pervasive discourse of reaction online. While it may appear as though differentiating between humans and bots is an increasingly straightforward and dependable technical operation, the evolution of CAPTCHA suggests otherwise. The means and the ends associated with confirming one’s humanity on the internet have changed significantly, but earning a place as a valid participant in online discourse has never been more fraught.
The origin of CAPTCHA can be traced to a now largely forgotten 1990s-era search engine, Alta Vista. Its unique value proposition at the time was a feature that allowed users to submit links for indexing and inclusion in search results. This made for a more participatory search experience, but it was vulnerable to hacking. Simple scripts could be written to automatically flood the search engine’s index with results for particular topics or products, allowing ideologues and hucksters to temporarily capture valuable search terms. Manually moderating the onslaught of submitted URLs was untenable, but a team of developers enlisted by Alta Vista’s parent company, the Digital Equipment Corporation, formulated an ingenious solution: Build an automated system that could detect and deny other automated systems from participating.
Simple enough in theory, this fix would require developing and implementing a system capable of automatically generating, administering, and evaluating a test that the system itself could not also automatically solve. Inspired by their office scanner, which had an optical character recognition feature that struggled to read smudged and skewed print, the D.E.C. team devised a system that could effectively impose and enforce a humans-only policy. It displayed a set of squiggly, offset characters and required the would-be link submitter to accurately retype them before they could submit a new link. This remedy, however, was provisional. OCR technology steadily improved, and the incentive to spam and scam search results increased with ballooning internet use. A cat-and-mouse game ensued between hackers and infosec engineers. Advancements in automation made by either party — whether in the making or the breaking of these puzzles — catalyzed the other to up the ante.
Around the same time, Yahoo, one of Alta Vista’s competitors, was dealing with a similar issue. Its spam-riddled chatrooms were in desperate need of sanitization. Looking for novel solutions, Yahoo sent its chief scientist to Carnegie Mellon University to deliver a job talk that would conveniently double as a brainstorming session with students and faculty. This visit captured the imagination of Luis von Ahn, a first-year doctoral student in computer science. For the remainder of his studies, culminating in an influential dissertation entitled “Human Computation,” von Ahn would gradually piece together a systematic approach to gamifying the simple tasks involved in proto-CAPTCHA systems like the one developed by Alta Vista. His core innovation was to harness the effort it took a user to validate their status and put it to use for other productive purposes. He called it “human processing power.”
Each CAPTCHA, von Ahn realized, required only a few seconds of work from each user and was therefore likely to slip beneath the perceptual threshold of activity that might register to them as work. He developed systems for agglomerating this mass of microwork and directing it toward large-scale computational problems. During his dissertation research, he created and launched several CAPTCHA-like games aimed at addressing longstanding computer science goals, including the identification of objects in images. One especially popular game, launched online in 2003, attracted thousands of players who tagged almost 300,000 unique images with 1.2 million labels in four months — a valuable cache for image-search platforms that would have been costly and time-consuming to produce by more traditional means. Google licensed the code for von Ahn’s image game and launched the Google Image Labeler in 2006. Within two years, more than 200,000 users had tried their hand at the gamified interface, collectively labeling more 50 million images. This enriched Google’s image search with invaluable human oversight, a marked improvement compared with the then-going alternative of scattershot algorithmic matching between search query and image file name.
CAPTCHAs are microcosms of an internet infrastructure that has been designed for the express purpose of capturing, pooling, and valorizing surplus attention of users
The bulk of von Ahn’s dissertation, however, was dedicated to CAPTCHA and to outlining different approaches to text-, graphic-, and audio-based puzzles that capitalized on the gap between human and machine perceptual acuity. By the time he defended his dissertation in 2004, CAPTCHAs were being solved at the scale of many millions per day, employed by search engines, free email clients, and e-commerce portals across the web. His approach illustrated concretely that the security vulnerabilities of online platforms could serve as a pretense for the extraction of surplus labor power. This insight compelled Google to acquire von Ahn’s next venture, reCAPTCHA, in 2009, which it has since used to enlist unwitting internet users in the transcription of books and newspapers and, more recently, the annotation of image datasets for use by the company’s artificial intelligence research unit. Above all, von Ahn’s work helped transform CAPTCHA from an online security checkpoint into a covert tollbooth. Thanks in part to these systems, to participate in online discourse would mean freely filling the coffers of big tech.
But the common, labor-driven critique of CAPTCHA and reCAPTCHA, while necessary, is insufficient. When a class action lawsuit was filed in 2015 against Google alleging unpaid wages for the work of solving reCAPTCHAs, it was dismissed without trial. The political-economic critique of these systems is based on a core truth, but one that is true of the internet in general: CAPTCHA and reCAPTCHA are not idiosyncratic engines of online exploitation but microcosms of an internet infrastructure that has been designed for the express purpose of capturing, pooling, and valorizing the surplus attention of users.
Moreover, critiques of CAPTCHA staked primarily to uncompensated labor fail to account for the recent changes made to its method of verification and authorization. As machines become increasingly adept at accurately deciphering text and identifying objects in images, these tasks become unreliable indicators that exclusively human work has taken place. Since 2013, Google has served fewer text and image puzzles, opting instead to actively screen users with backgrounded “risk analysis” protocols during browsing. This approach assesses each user visiting a web page through an undisclosed mix of passive and behavioral data that likely includes a user’s IP address and browser cookies as well as their mouse movements and click history. The mysterious standalone “I’m not a robot” checkbox that users now often encounter instead of a text- or image-based reCAPTCHA is largely a decoy: The necessary evidence has already been gathered by the time you click it. If this evidence suggests you pose a significant risk, only then will you be presented with a more traditional CAPTCHA puzzle, often involving low-resolution streetscape images.
With this update, the human-machine interplay crystallized in CAPTCHA has undergone a surprising reversal. “Today the distorted letters serve less as a test of humanity,” Google product manager Vinay Shet wrote in 2013, “and more as a medium of engagement to elicit a broad range of cues that characterize humans and bots.” In other words, there are no longer bots on one side and humans on the other, neatly classified along predetermined ontological lines by their respective capacities for perception, interpretation, and judgment. Instead there are only data producers: Some produce data that is more bot-like, and some produce data that is more human-like.
The standalone “I’m not a robot” checkbox that users often encounter is a decoy: The necessary evidence has already been gathered by the time you click it
By recasting humans and bots as both data producers first and foremost, CAPTCHAs have shifted their operative method of verification and validation. Where CAPTCHAs once created the conditions of indeterminacy (warped letters, skewed images, garbled audio, etc.) and prompted users for an interpretation, CAPTCHAs are now the interpreters of the fundamental indeterminacies exhibited by users (circuitous browser history, shaky cursor movements, etc.). From Alta Vista’s clunky widget to Google’s more sophisticated and surreptitious schemes, the onus of interpretation has been transferred from human to machine.
As CAPTCHAs have become more refined, ditching black-or-white determinations for a probabilistic spectrum of grays, human interlocutors, on social media platforms and elsewhere, have seemed to move in the opposite direction, increasingly eager to disparage their ideological opponents as “bots.” Liberals and conservatives alike are quick to blame bots for their respective bugbears, whether that be Russian disinformation or homegrown “fake news.” The artist and researcher Darius Kazemi has repeatedly critiqued these claims, demonstrating that bot hysteria is not only overblown but also mostly unfounded.
But those wielding “bot” as a pejorative seem largely agnostic about whether their targets are, in fact, automated systems simulating human behavior. Rather, crying “bot!” is a strategy for discrediting and dehumanizing others by reframing their conduct as fundamentally insincere, inauthentic, or enacted under false pretenses. The term intentionally denigrates rivals as members of an unthinking and automatic herd. This phenomenon is not exclusive to cyberspace: Some workers on the grocery-shopping platform Instacart have lately taken to disparaging unfamiliar colleagues encountered while on the job as “Bot Shoppers.” An outfit of teens in Arizona was recently enlisted to participate in conservative astroturfing campaigns on social media and given explicit instructions to mimic bots. After the scheme was uncovered, the group’s ringleader insisted that despite the bot-like behavior, this was “sincere political activism conducted by real people who passionately hold the beliefs they describe online.”
As the history of CAPTCHA demonstrates, no method of sorting and classifying is without political implications, but the specific methods that are implemented differ in meaningful ways and therefore lead to distinctive outcomes. Whereas CAPTCHAs once purported to test for something universal, shared by all human users, the new paradigm is one that looks for deviation from some probabilistically determined norm. The former was ripe for soliciting labor and capturing value at scale, as exemplified in von Ahn’s framework of “human processing power.” The latter, however, is much better equipped for teasing out and reifying normative differences between groups of users.
It’s not difficult, therefore, to imagine a near future internet in which CAPTCHAs are deployed not to prevent automated agents from corrupting systems but instead to validate users on ideological or political grounds. Paywalls and other content barriers could be augmented with CAPTCHAs that don’t ask you to contribute time or money but instead demand that you verify your worldview. You’re presented with a smiling woman unloading a dishwasher: Select the correct squares and you can gain access to a men’s rights subreddit. You’re presented with a grid of cops: Select the correct squares to view meeting details for a local abolitionist group. Other signifiers, superficially anodyne but charged with subterranean meaning — the “OK” hand gesture, a red rose, a cartoon frog, a corncob, etc. — already serve as semiotic fulcrums for the selective partitioning of online discourse.
Platforms eager to fully automate their content moderation systems could theoretically systematize this method of politicized interpretation, just as von Ahn did with his gamified microwork interfaces. But unlike the select-all-traffic-signs tasks codified in reCAPTCHA, these more speculative exercises in interpretation would prove useless as training data. They couldn’t help artificial intelligence systems determine the exact threshold at which irony flips into harm, for example, because there is no threshold. Distinguishing ironic content from harmful content is a judgment that depends upon contextual factors that humans are themselves unable to fully articulate and therefore are unable to fully automate. This is because context does not pre-exist judgment but is what the act of judgment helps produce and sustain. If it weren’t, what counts as context would be universal, unchanging, and apolitical, which we know is not the case.
Platforms already do a good job of sorting users according to their chosen contextual commitments and sustaining isolated enclaves of curated content. But the internet’s political economy of attention necessarily incentivizes and valorizes the productive friction that occurs when incompatible spheres come into contact with one another. Content that inspires exponentially more content — as when a tweet gets ratioed — is grist for the mill. That users have gradually internalized this structural incentive, becoming bot-like in their dogged pursuit of engagement, is unsurprising.
Indeed, the ongoing bot panic seems to consist mostly of humans calling out other humans for appearing to respond to this incentive. This logic implies that only a “bot” — something automatic and without agency, whether this bot be human, software, or some combination thereof — would so willingly provide fuel for the internet’s engines of discontent. But this diagnosis is predicated on wishful thinking, whether that be nostalgia for an internet that never existed or optimism for an internet that will never exist. It’s misguided not because the internet has always been and will always be populated by bots, but because it has always been and will always be populated by humans.
The journalist Lili Loofbourow recently declared that “bad faith is the condition of the modern internet.” Bad faith, in Jean-Paul Sartre’s definitive formulation, is a particularly potent form of inauthenticity that fully encompasses those captured by it. Bad faith actors willfully lose sight of the stakes or the shared, discursive ground on which they might otherwise stand with their interlocutors. Put differently, bad faith is the refusal to account for context. In an online environment in which everyone and everything is first and foremost a vector of valuable data — as the latest reCAPTCHA system posits — bad faith is universal because all content can be divorced from its context.
Loofbourow singles out the poisonous slogan “All Lives Matter,” which coalesces around it a coterie of actors brought together by a winking naiveté of history, of intent, and of context. As with early CAPTCHA puzzles, this seemingly benign and agreeable phrase is meant to incite particular interpretations in order to filter interpreters by type — in this case, by political conviction. But these convictions remain shrouded and indeterminate without a sufficient account of their historical and material context. One of Sartre’s examples of bad faith was a waiter in a café who had been conditioned by capitalist modernity to perform the role of waiter so precisely and so eagerly that his very humanity is called into question. Sartre referred to this waiter as an “automaton.” If he were writing in 2020, it’s safe to assume he would have opted for “bot.”