In a story that should have surprised no one, Mark Bergen of Bloomberg reported this week on YouTube’s history of prioritizing scale and profit over the well-being of its users, harnessing what its own engineers apparently call “bad virality” to optimize for engagement. “The conundrum isn’t just that videos questioning the moon landing or the efficacy of vaccines are on YouTube,” Bergen writes. “The massive ‘library,’ generated by users with little editorial oversight, is bound to have untrue nonsense. Instead, YouTube’s problem is that it allows the nonsense to flourish. And, in some cases, through its powerful artificial intelligence system, it even provides the fuel that lets it spread.”
This is basically the same point Zeynep Tufekci noted in this New York Times piece from a year ago about YouTube’s role in online radicalization: “It seems as if you are never ‘hard core’ enough for YouTube’s recommendation algorithm. It promotes, recommends and disseminates videos in a manner that appears to constantly up the stakes.” Tufekci went on to claim that “this is not because a cabal of YouTube engineers is plotting to drive the world off a cliff” but because of “the nexus of artificial intelligence and Google’s business model.” Alas, the news in Bergen’s story is that it was both: The engineers were plotting to drive the world off of the cliff because they knew that was what the business model demanded. “Conversations with over twenty people who work at, or recently left, YouTube reveal a corporate leadership unable or unwilling to act on these internal alarms for fear of throttling engagement,” Bergen reports. As Charlie Warzel notes in this New York Times op-ed, this is not just a problem with YouTube but with Facebook and Twitter and basically any other platform with a business model that sees content as a means of harvesting attention rather than an end in itself.
When you combine a radical agnosticism toward content with maximalist approach to amassing it, you end up with the kind of thing detailed in this Smarter Every Day episode (which, be forewarned, turns into a VPN sales pitch): automated content production on the model of spam, a futile arms race trying to contain it, and elaborate schemes to goose engagement metrics. The host, Destin Sandlin, calls this “artificial engagement,” but I think that term is a red herring: It legitimizes the idea that there can be something “real” and “human” about measuring attention in a system that is fundamentally indifferent to content. If it doesn’t matter what content does to people other than make them click, then why should a human view count more than a nonhuman one? Why should we let what advertisers think is necessary dictate what is seen as real and what is seen as fake? Advertisers are purposely trying to manipulate viewers; why, then, should viewers be prohibited from tricking advertisers?
Attention metrics aren’t “natural”; there is nothing about attention that is intrinsically measurable. The effort to impose metrics on attention is an attempt to control it, to make it exploitable. Marketers are not inherently entitled to monopolize those efforts just because historically their industry has been the one to most successfully monetize them.
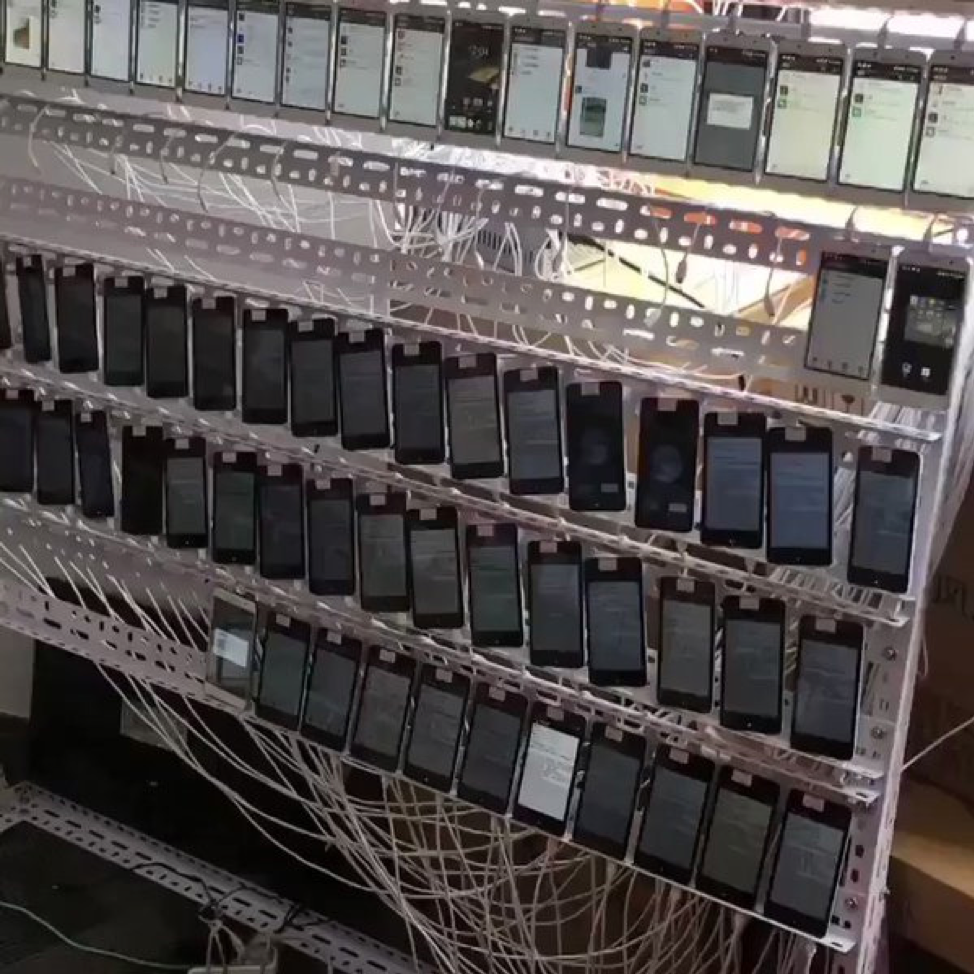
The humans who build “artificial engagement” machines like the one above are real humans, their efforts are not fake. They are trying to stake their own claim on how attention is measured and monetized. It’s not noble, but it’s not “artificial,” any more than “likes” are intrinsically “real,” no matter how they are produced. In other words, if you are going to take any of the measurements slapped on to social behavior as “real,” then you have to accept the reality of the efforts to game them as well.
What struck me mainly about the Smarter Every Day video was the section between minutes 11 and 13 that details how massive volumes of videos can be produced automatically (by companies like Wochit, which claims it makes nearly 60,000 videos a day) to spam YouTube with clips. Sandlin wants to differentiate between good and bad uses for such automatic content generation, but it made me wonder if all content produced without active and direct human intention should be regarded as suspect, if merely because of the deluge of material you can unleash when you are willing to do without it. The problem, to go back to Bergen, is not just with YouTube’s algorithms but with the massiveness of YouTube’s “massive library” — as reprehensible as some of that content it is, it is the constant stream of material into the algorithms for processing that is ultimate problem.
As with click farms, there is a temptation to call this automatically generated material “fake” because it operates beyond the limits of the human capacity for attention. But the point is that these tactics don’t require attention as an input; they produce attention in a specific measurable form. Automated content is the most efficient way to make the largest amount of measured attention from the smallest amount of immeasurable attention. Again, this inevitably follows from YouTube’s fundamental indifference to content and complete investment in engagement. But none of it is “inauthentic” unless you regard following Silicon Valley’s rules to be the gold standard of authenticity.
But more important than whether or not the content is “authentic” is the sense of identity it produces in viewers. Human intentionality is extinguished as an input into YouTube’s algorithms (at the level of the individual video anyway) only to be re-created as an output. That is, the flood of videos made by machines produces a human consumer of them who becomes governed by their underlying logic — that consuming certain kinds of content accrues to a user’s identity and testifies to their political agency, their ability to seemingly affect the world.
Sandlin posits that “attacks” on YouTube’s algorithm are either financial (“create videos to extract ad revenue”) or ideological (“meant to sway public opinion … and perhaps even make people fight with each another”). But those can be condensed into one. For content makers, as for YouTube, the “ideology” is profit, and the method is a variant on “divide and conquer” — stratify audiences into opposing groups and help make sure their sense of loyalty is predicated on further content consumption.
What is most irritating about Sandlin’s video, though, is how he then blames viewers for “the flaw in your heart” that makes us susceptible to fear and tribalism rather than blaming YouTube’s engineers — and ultimately capitalism — for incentivizing conflict. Financial motivation is itself ideology. It’s incoherent to claim that some videos are made for bad political aims and others are made to make money: Making money in the way that YouTube’s structure permits entails embracing a specific ideology about attention that overrides any particular messages in any particular content. It all points to radicalization, as Tufekci noted: “Videos about jogging led to videos about running ultramarathons.” Whatever is fed through the engagement algorithm is automatically politicized.
The point is not that recommendation algorithms start pointing to more extreme content; it is that its presence incentivizes the creation of more extreme content, on the automated, industrialized scale implied by the existence of firms like Wochit. For any category of content that draws an audience, a more “viral” version will be created to take advantage of how the algorithm works. In New Dark Age, James Bridle explored this idea with respect to videos for children. Attention metrics direct the algorithm, which then directs content makers, and none of them seem to care where they end up (“industrialized nightmare production,” in Bridle’s view), as they take refuge in the idea that they are just giving the audience (in this case, preteens) what they “want.” In Bridle’s words, “neither the algorithms nor the intended audience care about meaning.”
In a sense, that is basically what “bad virality” is about: the subordination of “meaning” to a more general impulsivity. But that impulsivity aims toward antisocial, destructive, or hateful beliefs. Casey Newton defines bad virality as “YouTube’s unmatched ability take a piece of misinformation or hate speech and, using its opaque recommendation system, find it the widest possible audience.” In the Bloomberg story, Bergen sets up the definition of bad virality with this: “In the race to one billion hours, a formula emerged: Outrage equals attention. It’s one that people on the political fringes have easily exploited, said Brittan Heller, a fellow at Harvard University’s Carr Center. ‘They don’t know how the algorithm works,’ she said. ‘But they do know that the more outrageous the content is, the more views.’”
It’s striking to me, though, that no one seems to know why “outrageous” or “extreme” or “radical” content attracts more attention; it often tends to be taken for granted that outrageousness is just inherently more compelling. Warzel likens radicalizing content to informational fast food: “Human beings have many natural tendencies that need to be vigilantly monitored in the context of modern life. For example, our craving for fat, salt and sugar, which served us well when food was scarce, can lead us astray in an environment in which fat, salt and sugar are all too plentiful and heavily marketed to us. So too our natural curiosity about the unknown can lead us astray on a website that leads us too much in the direction of lies, hoaxes and misinformation. In effect, YouTube has created a restaurant that serves us increasingly sugary, fatty foods.” We just like how extreme content tastes.
Sandlin, for his part, fully exculpates YouTube: “If your first inclination is to be mad at YouTube right now in some kind of ‘outrage’, then you don’t get it,” he says with concerned condescension. “I know these engineers, they’re using all the math at their disposal to try to fix this,” he continues, as if that is clearly the most responsible response. Thank goodness! The engineers are using math to “fix” an ethical concern. Bergen’s article makes it clear that engineers optimize for what their bosses tell them to optimize for, and ethics has nothing to do with it.
Tufekci explains the appeal of extreme content by claiming a “rabbit hole effect”:
What we are witnessing is the computational exploitation of a natural human desire: to look “behind the curtain,” to dig deeper into something that engages us. As we click and click, we are carried along by the exciting sensation of uncovering more secrets and deeper truths. YouTube leads viewers down a rabbit hole of extremism, while Google racks up the ad sales.
But that is just blaming human nature again, which is no more illuminating than a tautology: People want just what they want. Machine learning, from this perspective, merely exploits an already present human tendency. But the darker possibility is that exposure to these capitalistic systems of attention management fosters these tendencies toward extremism, not only because this can make individuals feel more defined to themselves but also because divisive extremism is the most profitable kind of population for media companies to work with. (Just look at Fox News’s success at turning families against each other.) Bridle equivocates on this point a bit, pointing to “latent desires” at one point, but I’m more convinced by his argument that “automated reward systems like YouTube algorithms necessitate exploitation to sustain their revenue, encoding the worst aspects of rapacious, free market capitalism. No controls are possible without collapsing the system.”
That explains why YouTube executives of course did nothing about “bad virality.” Bad virality is the business model. When Newton writes that “extremism in all its forms is not a problem that YouTube can solve alone,” you have to wonder question whether they are even capable of perceiving it as a problem. It seems more likely that they see bad publicity as their real problem, much like Facebook does when they are confronted about similar concerns.
Extremism isn’t simply extracted from the human heart of darkness by neutral machine learning processes capable of uncovering our “true desires.” The desire for divisive content is generated by the environment in which it thrives. It inspires using attention metrics as a kind of proxy weapon in wars among rival factions, even as it seems to constitute a rabbit hole that testifies to the individual’s diligence, daring, or savvy. Platforms don’t want to build one big happy community. They want to make many smaller communities who all hate each other.