To draw attention to machine learning research, it apparently helps to tout it as “dangerous.” The research company OpenAI received a lot of media attention last week by refusing to release the latest iteration of its text-generating/language-predicting model, GPT-2, claiming it was too good at producing readable text on command. “Due to concerns about large language models being used to generate deceptive, biased, or abusive language at scale, we are only releasing a much smaller version of GPT-2 along with sampling code,” they wrote in a blog post. “We are not releasing the dataset, training code, or GPT-2 model weights.”
The researchers’ posture of threat readiness seemed more like hype for its model than a profound concern for society. The press coverage tended to solemnly repeat the researchers’ warnings before moving on to fun experiments like getting the AI to write a story about itself, or rewrite some Brexit stories, or spew out some Tolkien fan fiction.
While reporters were giddily recounting the possibilities, there was, as Axios noted, a “massive blowback” from other AI researchers, who accused OpenAI of pulling off a “media stunt.” At the Verge, James Vincent pointed out that it had become “a bit of a meme among AI researchers, who joked that they’ve had an amazing breakthrough in the lab, but the results were too dangerous to share at the moment.”
The model, trained on 8 million web pages sourced from Reddit, works by using that data to establish probabilities of what words are likely follow other words. Stanford University researcher Hugh Zhang notes that the model, like many others, is good at producing sentences but bad at establishing continuity between them, and argues that “none of the samples shown by OpenAI are at a level where they can be directly misused by a malicious agent.” I’m not sure how he could possibly make that blanket statement — there are lots of ways to be malicious that don’t rely on fully coherent prose — but it’s also not clear what would make computer-generated text inherently more dangerous or “fake” than the massive amounts of text humans produce in bad faith. The OpenAI researchers warn that their tool could be used to “generate misleading news articles” (as if humans couldn’t already do that, and far more effectively), “impersonate others online” (as if this required massive amounts of coherent text to pull off), or “automate the production of abusive or faked content to post on social media” (as if what people do on social media isn’t already often contrived or abusive).
It’s the “automate” part that seems new: What an AI model might uniquely be capable of is spam-like scale. As the researchers explain it, “large language models are becoming increasingly easy to steer towards scalable, customized, coherent text generation.” Its model could generate a lot of different, semi-passable texts on a lot of different topics without human intervention — a million different junk emails fine-tuned to ensnare a million different people, in an enhanced form of targeted advertising. It might seem as though some actual other person cared enough about you to single you out, but no one is speaking — it’s more that you are seen as generic enough as an audience to receive the persuasive efforts of a nonthinking nonperson. Just as algorithmic ad auctions use data about you to constitute a rough approximation of who you are to suit its purposes — a identity governed by estimates of your demographic traits and interests — the AI text generator could posit who you might be and weight its model accordingly: probable text for a probable person.
Another way the model’s mass of text could be used is to populate comment threads, social media feeds, and review sites with posts that back a particular opinion or point of view to make it seem more prominent or popular than it is. Or the texts could simply overwhelm those systems with so much garbage that the human-written posts become hard to uncover or trust. It could also make all other reviews and comments seem suspect, as if they too were all just algorithmic nonsense. It could convert everything around it to dummy text.
But this points not to the danger of newly powerful AI so much as the vulnerability of platforms without editors. The concern with synthetic text is not that it will convince anyone that its content is worth considering, or that “fake” ideas will seem real. The places prone to hosting this kind of text are content agnostic anyway. Science Alert reported that GPT-2 can “generate random but convincing gibberish,” as if that is all anyone expects from a block of text anyway. (It’s an apt description of most of my writing.) Lots of text is no longer designed to be read but instead serves as a signifier of engagement or human presence, even as the words themselves are parsed and aggregated by machines rather than interpreted by human readers.
The worry over AI-generated text is similar to the fears about fake profiles and fake likes and fake page views and so on: It makes it seem as though there has been trackable human effort where there wasn’t any. Because quasi-coherent text has been nearly impossible for machines to make, it has stood as an imprimatur of an actual human taking the effort to express actual thoughts, even (or especially) when no corresponding human effort has been made to read it. Blocks of readable text, regardless of what they say, conveyed the all-important signal of human attention. So the issue with AI-generated text is less a moral or epistemological concern than a commercial one. Accurately measuring human attention is important for brokering its sale, not for establishing “truth.”
When platforms prioritize scale and volume of engagement, soliciting as much text as possible from anyone, they can’t abide silence and must be willing to publish as much random but convincing gibberish as is provided to them, while pretending it’s all well-intentioned. But a poorly guarded system inevitably rewards bad actors (like the AI-wielding miscreants who would theoretically flood these systems with “fakes”). Their success reshapes how everyone participates on the platform: Once anyone games a system fueled by engagement metrics and feedback, everyone has to. Since the system is indifferent to content, only tactics matter, and nothing prevents the “conversation” from sinking to the level of its most bad-faith participant.
In such discourse environments, where metadata rather than substance dictates the efficacy of communication, it’s easy to see how human-produced messages could be mechanized to the point where they could become indistinguishable from messages produced probabilistically by machines. This is the netherworld Jame Bridle writes about in New Dark Age, where search-engine optimization, financial incentives, and algorithmic feedback loops drive the creation of bizarre, repetitious content that functions outside human notions of coherence.
AI-generated text may have the effect of further driving human discourse down to its level in more environments, as it reveals how those places are also indifferent to content and prioritize other kinds of signals. The machine’s efforts at text may start to “seem more human” only because its implementation has assisted in making human behavior itself more mechanical — a point frequently made about AI in general. AI’s approximations of human behavior are so convenient and efficient, so much easier for sociotechnical systems to work with than people’s actual behavior, that it is elevated as ideal, or at least an achievable norm. We become like machines to talk to the machines that run phone banks and help lines. We order sandwiches by pressing buttons on a touchscreen, working our way through a flowchart like any other good algorithm. We fill in the blanks of emails with predictive text and pass it off as our own.
AI texts are post-interpretive: They dispense with what we used to call “the rhetorical situation,” in which meaning emerges from who is writing what to whom and why. Back when I was teaching freshman composition classes, I was trained to draw a triangle on the board to illustrate this for students; at the points were speaker, message, and audience. Here’s a more ornate version of it that looks a little more Alistair Crowley than Aristotle:
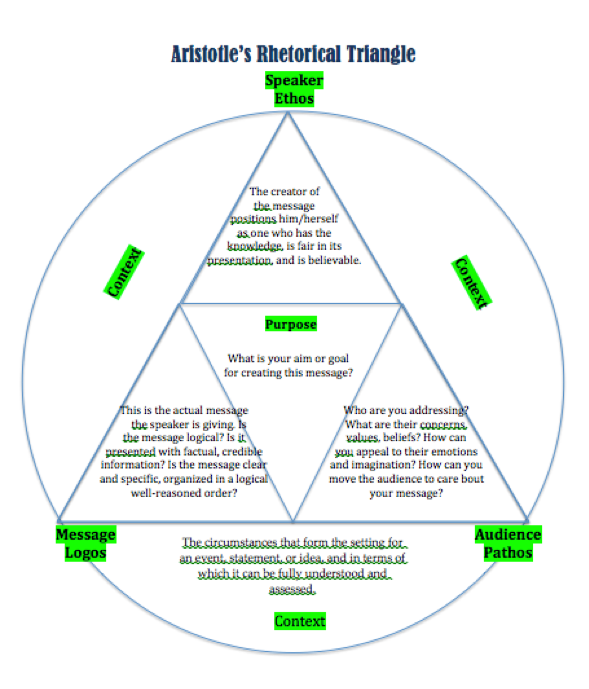
“Textual deepfakes” proclaim the rhetorical situation essentially irrelevant. They undermine the relation between text and meaning, subordinating it to the instrumental efficiency of working with blocks of text as so many ones and zeros. What are words for when no one listens anymore?
From the machine-learning perspective, meaning doesn’t necessarily have to emerge from one human interpreting what another human has put into language; it can instead be simulated through a brute-force analysis of words as nothing more than positional counters. It’s not important what people say or what they understand, but what they do — where their attention is. For media businesses, this is the only thing that means anything. AI-generated text is a problem only insofar as it muddies that water and makes it so that coherent prose can no longer be used as a proxy for attention. Hidden, then, in the concern over “fake posts” is a corollary belief that everyone is obliged to have their attention captured and measured, and that paying attention is the only meaningful form of speech. Clicks are all that’s left.
If AI-generated text devalues every point of the rhetorical triangle, replacing it all with content-free weighted probabilities, then something similar is at work in another AI-related project that has received a lot of recent attention: thispersondoesnotexist.com. Every time you load this site, you see a different AI-generated face, and you can look at a “fake” person who is the residue of millions of faces fed into a generative adversarial network.
The creator of the site, Philip Wang, told Motherboard, “Most people do not understand how good AIs will be at synthesizing images in the future,” which seems to frame the project as a kind of vague warning. It also frames AI as the agent in this rather than its programmers, as if AI wants to learn how to deceive us for its own reasons and this research is not being driven by a range of commercial interests.
Thispersondoesnotexist.com is effective at sidelining the political economy behind the technology powering it. It just shows us a person who seems to have been captured in a spontaneous snapshot. An aura of innocence seems to hang over them; they are not in selfie mode but are casual and apparently unselfconscious — which makes sense, of course, because there is no consciousness. But each new “person” that’s called up, the endless litany, conveys the idea that simply having a face is not enough to make you human anymore. Just as AI-generated text calls into question the human presence behind any piece of writing, AI-generated faces could have a similar effect on human faces, undermining their sanctity. Levinas’s idea that a face signifies living presence and elicits ethical behavior, an obligation to the other, becomes almost nonsensical. Rather than obligation, what I feel when looking at thispersondoesnotexist.com is idle curiosity, a compulsion to erase the face I am looking at to see a new one. Each new face doesn’t require anything more than another spin of the wheel.
The generated face severs the intuitive connection between faces and lived identities. A convincingly plausible visage doesn’t need any heritage, any life story; it’s just a matter of averaging images together. It feels like this is preparing the way for a more fully fledged nonperson whom we’ll be invited to treat with the same instrumentalism, a means to whatever whimsical ends we might come up with. It’s further training for seeing people in general as nonplayer characters, under the assumption that they might very well just be generated and programmed.
To communicate, these AI projects tell us, nothing needs to be intended or meant by anyone; there just needs to be probabilities. The newly generated faces or paragraphs derived from the existing datasets of faces and texts that; at some point no additional examples will be required to train the models. There is no reason to think there is anything special about your face or the sentences you wrote. They may have a certain percentage match with what the model would have come up with, or they may fall short. But it is just another probable face, another plausible piece of writing, or not. The world, or the model of it, is already essentially complete without it.
In the past few columns, I wrote a lot about how predictive analytics prescribe identity or constitute subjectivity — how algorithmic recommendation tries to posit who we are and shape us in terms of its capabilities. Those systems work to make living people into “fakes.” The generative AI projects do something like the inverse: They make an identity from scratch and posit it as sufficiently real. It suggests that whole selves could be like the nonexistent faces — they could appear fresh, without a lived history of choices and experiences, simply generated from data sets, training code, and model weights.
The self, rather than being something essential or evolving, could come to seem probabilistic — reconstituted moment by moment, with no clear causal links between each iteration, just like how the OpenAI model treats words when it generates its sentences. Rather than fashioning a self through agency and intention, through trying to make things happen, a self could be understood as fully structured, not by society so much as the data collected about it. Such a self would be both merely probable and fully real at the same time. The self and its simulation would merge.
That seems like the state of selfhood under conditions of algorithmic control. All day everyday, as we encounter the various algorithmic systems that populate our feeds and program the ads we see and so on, our identity is calculated and deployed based on the requirements of the system, our attributes generated on the spot like Dungeons & Dragons characters. We are constituted as a set of probabilities and valued accordingly; then when the encounter is over, that single-serving identity is discarded.
Whatever happens in those confrontations feeds opaquely into the calculations for reconstituting identity the next time, which could be any sort of situation in which someone want to judge or nudge you. They will be able to roll the dice and see what sort of person you are. The continuity that once seemed to underwrite the self can be cast aside. What we’ve done will only vaguely filter into who we become. Our experiences will accumulate only to disintegrate in larger pools of data, training models for other moments and other selves.