During the pandemic, the everyday significance of modeling — data-driven representations of reality designed to inform planning — became inescapable. We viewed our plans, fears, and desires through the lens of statistical aggregates: Infection-rate graphs became representations not only of the virus’s spread but also of shattered plans, anxieties about lockdowns, concern for the fate of our communities.
But as epidemiological models became more influential, their implications were revealed as anything but absolute. One model, the Recidiviz Covid-19 Model for Incarceration, predicted high infection rates in prisons and consequently overburdened hospitals. While these predictions were used as the basis to release some prisoners early, the model has also been cited by those seeking to incorporate more data-driven surveillance technologies into prison management — a trend new AI startups like Blue Prism and Staqu are eager to get in on. Thus the same model supports both the call to downsize prisons and the demand to expand their operations, even as both can claim a focus on flattening the curve.
Impersonal, large-scale coordination by models can seem like an escape from subjective politics. It is rooted in a longstanding desire to streamline political thought
If insights from the same model can be used to justify wildly divergent interventions and political decisions, what should we make of the philosophical idea that data-driven modeling can liberate decision-making from politics? Advocates of algorithmic governance argue as though the “facts,” if enough are gathered and made efficacious, will disclose the “rational and equitable” response to existing conditions. And if existing models seem to offer ambiguous results, they might argue, it just means surveillance and technological intervention hasn’t been thorough enough.
But does a commitment to the facts really warrant ignoring concerns about individual privacy and agency? Many started to ask this question as new systems for contact tracing and penalizing violations of social distancing recommendations were proposed to fight the coronavirus. And does having enough “facts” really ensure that these systems can be considered unambiguously just? Even the crime-prediction platform PredPol, abandoned by the Los Angeles Police Department when critics and activists debunked its pseudo-scientific theories of criminal behavior and detailed its biases, jumped on the opportunity to rebrand itself as an epidemiological tool, a way to predict coronavirus spread and enforce targeted lockdowns. After all, it had already modeled crime as a “contagion-like process” — why not an actual contagion?
The ethics and effects of interventions depend not only on facts in themselves, but also on how facts are construed — and on what patterns of organization, existing or speculative, they are mobilized to justify. Yet the idea persists that data collection and fact finding should override concerns about surveillance, and not only in the most technocratic circles and policy think tanks. It also has defenders in the world of design theory and political philosophy. Benjamin Bratton, known for his theory of global geopolitics as an arrangement of computational technologies he calls “the Stack,” sees in data-driven modeling the only political rationality capable of responding to difficult social and environmental problems like pandemics and climate change. In his latest book, The Revenge of the Real: Politics for a Post-Pandemic World, he argues that expansive models — enabled by what he theorizes as “planetary-scale computation” — can transcend individualistic perspectives and politics and thereby inaugurate a more inclusive and objective regime of governance. Against a politically fragmented world of polarized opinions and subjective beliefs, these models, Bratton claims, would unite politics and logistics under a common representation of the world. In his view, this makes longstanding social concerns about personal privacy and freedom comparatively irrelevant and those who continue to raise them irrational.
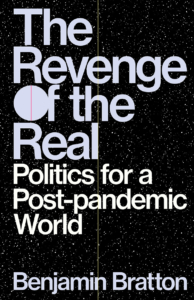
We should attend to this kind of reasoning carefully because it appeals to many who are seeking a decisive approach to dealing with the world’s epidemiological, climatological, and political ails, which seem insurmountably vast and impervious to tried political strategies. The prospect of impersonal, large-scale coordination by models may seem especially attractive when viewed from within a liberal political imaginary, where the dominant political value is a preoccupation with defending individual “rights,” rather than supporting the livelihood of others or the natural environment. An appeal to the objectivity of models can seem like an escape from subjective politics.
This idea has its roots in a longstanding desire to streamline political thought through an appeal to technical principles. It can be traced back at least to Auguste Comte’s 19th century positivism and his idea of developing a “social physics” that could account for social behavior with a set of fixed, universal laws. Later in the 19th century the term was taken up by statisticians, covering their racist criminological and sociological theories with a veneer of data. Now the premise of social physics lives on in everything from MIT professor Alex Pentland’s work on modeling human crowd behavior to the dreams of technocrats like Mark Zuckerberg, whose quest for “a fundamental mathematical law underlying human social relationships” is in conspicuous alignment with Facebook’s pursuit and implementation of a “social graph.”
But a “social physics” approach that broadens the horizon of computational modeling can seem appealing to more than just tech-company zealots. In more disinterested hands, a compelling political case can be made that it is the only viable way forward in a world out of control, marked by demagoguery and mistrust. As the hopes for modeling gain momentum, it will become increasingly important to articulate clearly its shortcomings, inconsistencies, and dangers.
The technological infrastructure needed for “planetary-scale computation” and all-encompassing models doesn’t need to be invented from scratch. According to Bratton, it’s everywhere already: diverse computing devices, sensors for data collection, and users across the world are networked together in ways that afford humanity the ability to sense, model, and understand itself — everything from logistical supply chains to climate modeling. The planetary network of computation has already spawned well-documented abuses of overreaching data collection and harmful side effects of automating human decisions, but these are not Bratton’s concern. In his view, planetary-scale computation’s core architecture is practical and effective; it’s just that its current uses are misplaced and human, geared more toward tasks like collecting data to target individuals with ads than intervening in climate change.
Bratton sees logistical platforms like Amazon and China’s Belt and Road Initiative as precedents for what planetary-scale computation should become: a data collection and analysis system that doesn’t merely try to optimize attention but instead makes services more efficient. Epidemiological modeling has become another precedent: The pandemic, Bratton suggests, has pushed us to develop models through which we perceive ourselves “not as self-contained individuals entering into contractual relationships, but as a population of contagion nodes and vectors.” That is, the coronavirus — by infecting people irrespective of their subjective intentions, politics, and accords — demonstrated that objective, biological forces always have the last say. This challenges us to recognize what Bratton calls the “ethics of being an object”: the importance of acknowledging ourselves as biological things that can harm other things regardless of our subjective values and intentions. Rather than resist expanding surveillance measures, this “epidemiological reality,” Bratton argues, should have prompted us to forgo personal privacy and agency in favor of being accounted for by planetary-scale computing systems and modeling.
Epidemiological modeling exemplifies what Michel Foucault described as “biopolitics”: a strategy of governance that uses statistical aggregates to represent the health of human populations at scale, enabling authorities to identify how individuals deviate from a “norm.” Often, Foucault is read as critiquing such strategies for extending regimes of normalization, but Bratton believes that there can be a “positive biopolitics” that demonstrates what population-scale abstractions provide to medical care over what they confer to political power, as though the two could be disentangled.
Law enforcement agencies consistently appeal the notion that surveillance can work as a positive form of inclusion
For Foucault, subjectivity is a site and strategy of power. In other words, power exercises itself through shaping how we understand who we are. Unlike traditional juridical power in which a sovereign or state governs by decrees and prohibitions, what Foucault calls “biopower” can “dispose” human behavior to certain ends without needing the state to formally categorize or criminalize it. This account of biopower, too, is usually seen as a critique: That biopower coerces through incentives and data rather than through observable contracts and conspicuous acts of violence demonstrates its insidiousness. By operating through algorithms that manipulate human environments and attention, biopower can nudge human behavior without issuing explicit commands or receiving considered consent.
For Bratton, this abrogation of personal agency appears as a potential advantage, putting the “ethics of being an object” into practice. Operationalizing population-scale Covid infection data, for example, could override individualistic myopia as well as clumsy conventional forms of state authority, which resorted to blanket lockdown measures only because they lacked the jurisdiction and capacity for more targeted measures. After all, Bratton argues, “Those in socio-economic positions that prevent them from receiving the medical care they need may be less concerned about the psychological insult of being treated like an object by medical abstraction than they are about the real personal danger of not being treated at all.” They would rather be objectified than neglected entirely.
In practice, however, biopower tends to be not either–or but both: Marginalized groups are both surveilled and neglected, and neglect is used as a pretense for surveilling them more. For Foucault, distributed biopolitical governance is no less partial and coercive than traditional sovereign power; moreover in his view these forms of power do not exclude one another.
Biopolitical logic can be used as the pretense for exercising repressive sovereign power, as when the city of Portland broke up encampments of the unhoused for violating social distancing, gathering, and accessibility guidelines. And biopolitics can provide the basis for means-tested welfare policies, withholding benefits from targeted groups on the basis of objectifying abstractions. As cities in Texas experiment with technologies for registering houseless people on a blockchain to track the aid they receive, those who opt out of such surveillance may find themselves without access to essential services. This practice may appear more “efficient” by some ostensibly objective standard precisely because it leverages existing distributions of power. As these examples suggest, an appeal to biopolitics does not absolve us from the ethical quandaries of how subjects are represented and manipulated, even when such abstractions are carried out with the intent of distributing aid or providing resources to those in need.
If data-driven governance aims to influence human behavior at scale, it must eventually consider carefully the ethics of how power configures subjectivity and how this complicates distinctions between objective and subjective reason. As Foucault argued, this does not mean we should return to the model of human rights and subjectivity provided by juridical power, where governance is organized by individual subjects who enter into contractual relations with one another. Rather it demonstrates that such a model is inadequate to contemporary configurations of power and that new models are needed.
But the inadequacies of liberalism should not be taken to invalidate all critics of large-scale computational platforms. Bratton contends that “anti-surveillance” critique, broadly conceived, constrains discussions of technology to individualistic concerns over the rights and privacy of liberal subjects, effectively refusing to acknowledge how a lack of surveillance leaves certain communities, places, and processes unfairly unaccounted for. That is, critics of surveillance are ultimately arguing against what Bratton sees as inclusivity.
For Bratton, inclusivity has less to do with participation in governance than with “quantitative inclusion”: having information about people accounted for in metrics, data, and models. Subjects should embrace the “ethics of being an object” and thereby renounce any decision about whether they should be included in systems that they don’t have a practical say in developing.
By this view, the more inclusive model is the one that incorporates more diverse people within the scope of its calculations, ensuring their “right and responsibility to be counted.” To the degree that individuals refuse to be modeled, they are to blame for the models’ failures to be comprehensive enough to represent the world accurately and equitably — and not the extensive history of inequality in computing design and use. From Yarden Katz’s history of the white supremacist disposition of AI in Artificial Whiteness, through Virginia Eubanks’ investigation of discriminatory welfare algorithms in Automating Inequality, to Safiya Noble’s analysis of Google Search’s racial biases in Algorithms of Oppression, we find these inequalities at every step of the computing pipeline.
By viewing society in terms of networks of harm, it becomes easier to view solutions to all our problems in terms of data networks
The notion that surveillance can work as a positive form of inclusion is not new. Law enforcement agencies consistently appeal to it under a variety of names (evidence-based policing, information-driven policing, community policing) as a way to make neglected communities safer, as if greater scrutiny over their behaviors is what these communities need most. This data-based inclusivity — in which data about the number of times that communities commit crimes, call the police, or violate building codes are included in policing models — is different than inclusivity in decision-making, let alone inclusion in socially privileged groups. This is a data-driven iteration of what Keeanga-Yamahtta Taylor has called “predatory inclusion” with respect to the real estate industry’s efforts to mask housing inequality and segregation.
While surveillance may be branded like cellular network coverage, as though it can reach greater populations to provide them with better access to various services, it turns out to be demographically slanted. Increased surveillance is not equivalent to more equitable treatment but an expression of its opposite. This is characteristic of most appeals to data-driven modeling in governance: Maximum “inclusivity” and representation in data that ultimately enables more stringent conditions for gatekeeping who can participate in the design of decision-making processes. Bratton, for instance, gives precedence to “objective” accounts of data and algorithms over “subjective” accounts of their harms and is dismissive of activists who express concerns over such issues as “algorithmic bias” without adhering to technical definitions of algorithms. To be sure, the term “algorithmic bias” can be used as a ready-to-hand watchword for algorithm critique that precludes sustained reflection. But initiating an algorithm naming contest — Bratton challenges us with a list so we know where he stands: “A* search algorithm? Fast Fourier transform? Gradient descent?” — ultimately supports a regime of exclusivity based on technical competency, barring certain people and ways of knowing from discussions about technology ethics.
The argument for data-driven governance takes biopolitical control and mandatory inclusion as fundamentally necessary and rational, a view which follows from seeing data-driven models as more explicit and unambiguous in their aims than human subjects. Once designed, models march toward their preconceived purpose according to an objective logic that adapts to contingencies. Bratton thus maintains that there is nothing inherent in planetary-scale computation that necessarily disposes it to detrimental uses; it is basically neutral, as though computation at scale offered no concrete and practical affordances to power. The harmful uses of computation with which we are increasingly familiar are instead simply irrational applications of the technology against its grain.
But if planetary-scale computation is currently limited by the irrational social and cultural uses to which it is put, this doesn’t bode well for using this very same technology to transcend the politics that yield those apparent irrationalities. When Bratton presents the racial profiling and modeling of individual consumers that occurs on social media platforms as simply impractical uses of computation rather than practical tactics of power that planetary-scale computation specifically enables, he elides the entrenched material constraints and incentives of technology design that contribute to these practices in the first place. From communication platforms subsidized by user data to the biopolitical use of this data to analyze familial networks as criminal gangs, racial profiling and consumer behavior modeling aren’t simply misuses of computation, but affordances of it.
Without regard for these conditions of computing design and use, Bratton’s work reinforces the notion that computing applications are fungible: Social media technology can be applied to promoting social distancing instead of optimizing consumption; facial recognition technology can be applied to detecting infection instead of racial profiling; surveillance platforms can rebrand from crime prediction to infection modeling — and vice versa. After all, Bratton insists that social relations and ethics have an intrinsically epidemiological basis. By viewing society in terms of networks of harm, it becomes easier to view solutions to all our problems in terms of data networks, surveillance coverage, and interactions among distributed people and devices that must be regulated. Repurposing the existing architecture of planetary-scale computation to solving these problems becomes not a matter of on-the-ground “subjective” criticism of the harms it has caused but an unwavering commitment to its “suppressed positive potential.”
Throughout his work, Bratton encourages the design of automated mechanisms for governance that “can be repeated again and again, without the trouble of new deliberation.” By imbuing human decisions into computational systems and then designing them to respond to the environment dynamically, we can automate extraneous political discussions away. As an example, Bratton points to the water faucet: It automates political decisions like “where should the water come from, and for whom should it now come,” simply making water available to whoever turns the knob.
But this basic example obscures fundamental questions about technical interventions: Who designs them? Who decides what they ultimately do? How could we responsibly account for their unintended consequences? Mistakes are an inherent aspect of any scientific process or political intervention. Appeals to data-driven modeling in governance must take into consideration how data-driven calculations, no matter how descriptive or statistically accurate in controlled experiments, can lead to unanticipated problems.
For Bratton, models should not purport to know everything about the world. Instead, they must be carefully designed to abstract features from it, including and excluding details in the service of a particular objective. Since modeling cannot hope for perfect omniscience and omnipotence, it should be based instead on the specification of “heuristics” that reflect the practical, scoped tasks that we want modeling to achieve. Bratton does not give an example, leaving us instead with some questions to consider for modeling: “Will this work? Is this model modeling what really matters? Will this have the intended effect?”
This inevitably introduces subjectivity into modeling: What we choose to optimize, the values and measurements that we choose to attend to, are matters of subjective decision-making that cannot be comprehensively and automatically dictated by the “objective” data that we have at our disposal. This is an irreducibly political aspect of all appeals to modeling and objectivity in the real world.
The problem with planetary-scale computation is with scale itself. It fails to reckon with what we might call the deep time of facts
Philosophers associated with “neorationalism,” like Ray Brassier, Reza Negarestani, and Peter Wolfendale, theorize how this subjective limit could be overcome by models that interact with reality and learn to account for their own limitations automatically — a speculative version of machine learning algorithms. Bratton’s positive biopolitics too depends on such self-reflexive or recursive processes to decide and enforce “control over what matters.”
But climate models, for instance, cannot simply be mobilized to “act back upon the climate” without compromising the accuracy of their observations at scale. The computational logics of machine learning and recursion cannot shortcut the meticulous and contentious work of climate science. Effective climate models like the Geophysical Fluid Dynamics Laboratory Coupled Model are designed and validated by comparing their projections with concrete scientific observations in other domains and scales, requiring their manual refinement until they can hope to approximate large-scale phenomena to some degree of reliability. For these models to inform climate interventions would make that impossible.
A computational intervention that can recursively model the real-world consequences of its oversights remains a speculative fiction. Fantasies about such processes often fail to acknowledge how precisely information systems would have to be designed not simply to make informed observations about the most basic biological facts (like, say, the effects of masks on the probability of respiratory disease transmission) but also to account for site-specific contexts that reframe analysis of these facts, their social implications, and their tendency to change variably in response to targeted interventions (as when people try to “game the system”).
The problem with planetary-scale computation isn’t, as Bratton and other champions assert, that its potential is compromised by impractical applications, ideological criticisms, and a lack of recursive models. The problem is with scale itself. It fails to reckon with what we might call the deep time of facts: their historical heterogeneity, their capacity to change unpredictably in response to interventions, and their variations across geographical and cultural contexts. Even the historical unfolding of the internet, a symbol of large-scale technological integration across continents and timescales, shocked peoples and polities into diverse geopolitical positions that they are still reckoning with today. At each node of the internet — and in the territories that remain without access to it — the emergence of a planetary technical fact collided with heterogeneous cultural contexts to produce compounded layers of new facts that we are still unearthing.
This is one of the prescient insights from the field of design research and criticism called “postcolonial computing.” Against a tradition of design that seeks technological solutions for cultural problems, postcolonial computing recognizes how culture and technology use shape each other in dynamic interaction. It challenges the expectation that good intentions tend toward good interventions, particularly the more that they are backed by data, and the broader the scale they operate over. When projects proposed for people in other countries, demographics, or socio-economic conditions fail to acknowledge fundamental cultural differences and power relations, good intentions are often rendered obsolete. Today we continue to see how tech platforms built for scale, like the “decentralized” banking platforms designed to be implemented throughout countries in Africa and South America, prioritize their experiments over the people who will be subjected to their drastic changes and failures.
For postcolonial computing, understanding the impact of technological intervention requires a critical commitment to the local — a commitment that more “inclusive” coverage by data collection will never be able to supersede. A thorough commitment to the local is not an escape from acknowledging interdependencies between geopolitical territories, technological platforms, and subjective politics, but a grounded appreciation of how power maneuvers through scales of abstraction to ends that always have a local impact.
Experimentation in science and politics is vital, but its value has an important caveat. Just as scientific observations depend principally on site specificity, political experiments are most equitable when the people they affect have a stake in deciding them. At the basis of every technological intervention, no matter the facts used to justify it, is a subjective decision about what decisions can be made on behalf of others. Data-driven governance cannot automate these decisions away.