“Cringe” can seem like a self-explanatory phenomenon — a kind of reflex of revulsion toward some public show of awkwardness or cluelessness. The term emerged as online shorthand for a feeling that social media had made more common: “second-hand embarrassment,” as one UrbanDictionary contributor put it. But in recent years, as that site’s evolving definitions suggest, cringe has been increasingly associated with a sexualized shame. When I searched Instagram for the hashtag #cringe in early April 2022, for example, sex appeared as a clear unifying theme in what it denoted, from over-the-top expressions of desire to hypothetical genitals stuck in katsup bottles.
This suggests that “cringe” might serve as an index to how the regulation of sex, sexuality, and gender (i.e. cisheteronormativity) has shifted along with the technologies — of identification, of surveillance, of capture — that are necessary to carry it out. In a recent piece for the New Inquiry, Charlie Markbreiter argues that “cringe” has been mobilized against the increased visibility and relative inclusion of trans people in American life, “weaponized by the right as a form of social control.” But what makes it potent may be how it aligns with new modes of control that are implemented less in terms of fixed norms and more in terms of evolving probabilities.
“Cringe” is deployed as a kind of vibe that insists on an unassailable truth of the cringers’ deficit of positive feelings and the trans person’s responsibility for it
When a trans person is marked as “cringe,” it converts their non-cis-ness to a kind of social cost, a burden imposed on others that registers as their discomfort. Cringe marks individuals for exclusion from society’s care and protection not in terms of some sort of general deviation (i.e., the fact one is trans and not cis) but a subtler form of perceived nonalignment. It becomes capable of further subdividing trans people “into acceptable and surplus,” Markbreiter writes, on the basis of how their relation to their own transition strikes other people — how effective or plausible it seems. That is, “cringe” is deployed as a kind of vibe that avoids talk of broad norms yet insists on unassailable truth of the cringers’ deficit of positive feelings and the trans person’s supposed responsibility for it. In practice, this means that cringe is mainly applied to trans people without access to the resources (money, access, insurance, care) necessary to transition unobtrusively, via the sorts of medical procedures that generally lead to easier state and institutional recognition. This fuses social and economic marginalization while obfuscating the nature of their connection, such that trans people, as Markbreiter notes, can internalize a cringe response to their own predicament.
This places cringe squarely in the realm of what Michel Foucault called biopolitics, a form of power that uses statistical population models to identify “surplus” groups that can be targeted for reform or elimination. But it reflects a refinement of that power to accommodate new algorithmic modes of control.
Traditionally, populations have been excluded on the basis of norms, as illustrated by Francis Galton’s 19th century eugenics, or Richard Henderson and Charles Murray’s update of Galton in The Bell Curve. They argue that the distribution of intelligence in a population can be plotted as a bell curve — the form of Gaussian probabilistic statistics that Galton used to distinguish “eugenic” from “dysgenic” people. The bell curve, or normal distribution, establishes a normal range (the “bell” or the curve) for a variable such as IQ, which becomes the basis for corrective and exclusionary practices. For example, in the early-to-mid 20th century, the North Carolina Eugenics Board targeted “low IQ” individuals (who were also overwhelmingly from racial minorities) for forced sterilization. So it is that statistical distributions are converted into social relations. The past is made into a model of how the future is supposed to be.
Throughout the 20th century, this approach to probability served as the main justification for excluding people from the protections and entitlements granted to full members of society. However, the algorithms behind today’s tech and finance are mathematically different from that sort of statistical population modeling, relying on determining correlational probabilities rather than measured empirical distinctions. And because these algorithms are responsible for tasks ranging from vetting job applications to proctoring tests and surveilling workers to diagnosing medical conditions, they have very real power over the daily lives of average people.
In Revolutionary Mathematics: Artificial Intelligence, Statistics and the Logic of Capitalism, Justin Joque notes how today’s AI and machine learning algorithms don’t use a Gaussian model of probability (which tracks the past frequency of a variable in a population) but a Bayesian one (which fluidly calculates probabilities for future occurrences). “Instead of imagining and then defining some group that becomes the reference class that is being sampled from,” he writes, describing how norms are established, “Bayesian approaches imagine the researcher or the computer as an agent that continually gains more knowledge of the world.” That is, they adjust their models and predictions as they assimilate more data.
Like a bizarro version of feminist standpoint epistemology, contemporary algorithms calculate probability in terms of the orientation or belief of a situated subject: “Probability is,” as Joque puts it, “subjective.” Bayesian models sketch out an epistemic standpoint from which speculations can be made about future possibilities, everything from like what song I am likely to want to listen to next or whether someone should be granted parole based on the predicted likelihood of their recidivism.
As Bayesian techniques of modeling the world and behavior have been disseminated, they have changed the means of governance. It no longer mainly occurs at the census-like level of populations plotted on bell curves; it occurs through the algorithmic profiling of individuals, a technique that media studies scholar John Cheney-Lippold associates with “the soft biopolitics of control.” Rather than chart where members of a population fall in terms of a stably defined variable (e.g. where one scores on a standardized test), algorithmic approaches treat the variables themselves as evolving probabilities with respect to detected correlations within open-ended data sets. So, as Cheney-Lippold details, this process “uses statistical commonality models to determine one’s gender, race, or class in an automatic manner at the same time as it defines the actual meaning of gender, class, or race themselves.” For example, “gender” can be assigned to each particular individual within a population as a probability: Based on the data we have about their behavior, this subject is 74 percent likely to be male. Here, a data profile forms a standpoint from which algorithms can speculate about an individual’s probable gender identity while at the same time adjusting the boundaries of the category of “male” to reflect the perspective of this standpoint. The probability measures the degree to which the profile in question is aligned with what, from the algorithm’s perspective, counts as “male,” which is recalculated from instance to instance.
Like a bizarro version of feminist standpoint epistemology, contemporary algorithms calculate probability in terms of the orientation or belief of a situated subject
This is an entirely different way of mathematically modeling people, and a different means of control follows from it. If the welfare state used traditional probability to model populations, the new speculative math (as political theorist Melinda Cooper has argued in Life as Surplus) allows for the profile of specific people, to determine their capacities or possible impacts and evaluate whether they are ultimately productive or threatening. It’s less focused around norms (identifying outliers in a population and either ejecting them or trying to change them to fit into the bell curve) and more focused on legitimacy, identifying the resonance between a profiled subject and a situated observer as either productive or unproductive. A profile can be considered legitimate if it correlates or is aligned with whatever a specific algorithm has been trained to recognize as a tendency to positively contribute to the social status quo — i.e., if it gives off good vibes. Cringe is when it doesn’t.
For example, as a 2019 ProPublica report details, the TSA’s full-body scanners mark individual travelers out as potential threats by comparing a traveler’s body to an algorithm’s ever-shifting perspective on what a man’s or woman’s anatomical profile ought to look like, effectively singling out non-binary, gender non-conforming, and trans people. The algorithm, in this sense, cringes. In this process, the burden is placed on non-cis travelers to privately and resiliently overcome these impediments and continually try to align themselves with the scanner’s shifting norms. Travelers who cannot privately assume the costs of a legibly gendered body get singled out for extra police scrutiny. From the perspective of a society that criminalizes poverty, insufficient wealth is grounds for illegitimacy. At the same time the algorithmic judgments are disseminated throughout society to structure when others should feel cringe toward someone — sensitive to slighter deviations in what amounts to a never-ending audit of each other.
With the widespread adoption of proctoring software and platforms that surveil student email, messaging, and social media, schools — once one of Foucault’s key examples of normalizing institutions — are being remade into sites of algorithmic legitimation. As AI and ML algorithms transform our daily lives, making our homes smart and our media feeds personalized, they also fold us into the biopolitical regulation of legitimacy.
Norms, of course, are still around. But their power over people — especially white middle-class people — is waning with the rise of algorithmically shaped experience. This is reflected, for example, in debates about the future of queer activism. Because sexuality has been traditionally regulated and contested through norms, queer theory and activism have historically framed themselves as anti-normative practices. For most of the 20th century, queer people were represented as a statistically deviant population, so — as philosopher Ladelle McWhorter put it in the 2012 paper “Queer Economies” — “queer politics was about displacing the whole notion of identities constructed out of deviations and norms.” To be queer was to be against the norm; by breaking (gender) binaries, building chosen families, and refusing to follow the rhythms of so-called “adult” life, queer theory and activism sought to abolish norms.
But by the early 2010s, queer theorists recognized that sexuality was increasingly being regulated with tools other than norms and began to decouple queerness and antinormativity. As McWhorter puts it, “some formerly deviant homosexuals, for example, might be allowed to become the trendy (white, middle-class) gay couple next door — neither normal nor abnormal, just a variant within the general population.” As the parenthetical suggests, full personhood may still be denied someone on the basis of race or class, but some sexual norms were becoming less salient.
In the introduction a 2013 special issue of the feminist theory journal differences, editors Robyn Wiegman and Elizabeth A. Wilson claim “the possibility of a queer theory without antinormativity is both a necessary and timely pursuit.” Coincidentally, “normcore” — an elaboration of one vision of post-normativity — was defined in a trend report released the same year. “Normcore” embraced generic taste (khaki cargo pants, pumpkin spice lattes, etc.) as a putative rejection of having to constantly refine our human capital by proving our taste-making or trend-tracking capabilities. In some respects, normcore was a prescient throwback to (or nostalgia for) norms in the face of the rising power of algorithms. But the concept depended on aestheticizing a mainstream that has become more a myth than reality. Social media platforms, in their algorithmic personalization, epitomize an era in which vibes may shift, as they say, but there is no agreed-upon era-defining vibe, no standardized perspective toward them, just shifting probabilities and alignments. Norm in this context becomes merely a style, not a status — one more trend among many and not “normativity.” Whereas the queer antinormativity of the 20th century was a political strategy for intervening in the regulation and policing of sexuality, normcore-ish approaches to evading personalization lacks political punch because norms are the wrong target to subvert.
In the new biopolitical regime, belief,” “perspective,” or “vibe” function in place of norms to guide behavior. When terabytes of user data or the infinite variety of trends or styles threaten to overwhelm decision-makers, probability models intervene to present them with focused, actionable options — an orienting perspective, a standpoint from which some things come into focus as others are framed out. Recommendation algorithms, for example, help users sift through Amazon’s seemingly infinite array of products by highlighting products purchased by users with similar profiles or perspectives.
Take, for example, this image, which multiple websites use to explain how the process known as collaborative filtering works:
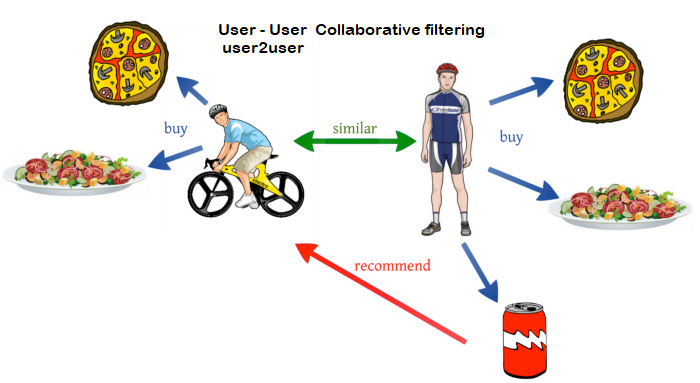
This diagram depicts two people with similar profiles. Since both bought a pizza and a garden salad but the person on the right also got a soda, the person on the left will be assumed to want a soda too. Probability here is not a frequency-based prediction but a spatial or directional one. This is what makes “perspective” and “vibe” such applicable terms for algorithmic governance.
If bell curves allowed for the comparison of individuals to the overall population to determine their supposed fitness, the new form of biopolitics finds correlations among data profiles to determine if an individual is headed in productive or unproductive directions. As Joque puts it, “everything now appears subjective, but this subjectivity is tied to a contract market, which in the end requires that all who arrive at market act objectively.” It doesn’t matter how normal or abnormal someone or something is, so long as it contributes to the patriarchal racial capitalist project of private property accumulation.
Normcore-ish approaches to evading personalization lacks political punch because norms are the wrong target to subvert
Whereas surplus populations have traditionally been marked out and penalized for their difference from the norm, in the new form of biopolitics the cumulative disadvantage of the traditional system makes members of those disadvantaged groups appear, from the perspective of these new “subjective” probability models, like bad risks unworthy of investment — i.e., as illegitimate. To take an example from Cooper’s Family Values, the difference between the married white gay couple celebrated on the cover of Time magazine and the demonized figure of the “welfare queen” lies not in the “normality” of their sexual behaviors but in their differing ability to privately assume the risks and costs of those behaviors: The couple can; the woman on public assistance does not. In Cooper’s terms, the couple’s relation is legitimate because it contributes to the patriarchal racial capitalist accumulation of private property whereas relying on public support is illegitimate because it does not.
From this perspective, it’s no surprise that trans scholars have been focusing on ideas of mutual aid and care rather than antinormativity. For example, DJs Eros Drew and Octo Octa have released a series of guides detailing how to DJ and how to set up a home studio. Modeled on practices of knowledge sharing in trans communities, these guides distribute knowledge that is traditionally gatekept both algorithmically (by the YouTube algorithm recommending explainer videos, for instance) and physically (by the ability to count on having safe clubbing experiences). Written in a welcoming and supportive tone, these e-zines both adopt the perspective that everyone deserves a chance to be a DJ and, as a system of knowledge distribution that is comparatively more equitable than the old boys club, help make that a reality. Ending on a note that emphasizes reciprocal knowledge exchange and supports creative risk-taking, the guide situates its vibe as an alternative to cringe’s policing of deservingness as a scarce resource. These are precisely the sorts of practices we will need to begin to help the people excluded by logics of legitimacy.