In the early 2000s, a new radio format emerged, playing a mix of pop and rock hits from the past several decades targeted at white adults. One could identify stations that adopted this approach by their formulaic name: some generic dude’s name plus “FM” (There was Jack FM, Bob FM, and so on). Part of the format was its faintly self-satisfied taglines delivered in a snarky, very obviously white-guy baritone (like Ron Livingstone’s character in Office Space, but deeper): “Playing what we want,” “’70s, ’80s, whatever,” and “True variety that rocks.”
At the time, this approach seemed rebellious and revolutionary — an independent “whatever” to radio industry orthodoxy — because, as Eric Weisbard notes in Top 40 Democracy, it defied a highly successful approach from the previous three decades: “the incorporation of genre by formats.” That is, formats, which describe a market demographic, had been largely grounded in musical genres, which describe a particular aesthetic. Jack FM’s “whatever” approach flouted traditional genre and format boundaries, conceiving of its audience instead in terms of what sociologists Richard Peterson and Roger Kern identified in 1996 as “omnivorous” cultural consumption. Whereas highbrow taste was conventionally defined by its exclusivity (you only like the most prestigious art), it is now defined by its flexibility (you like prestigious art and a mix of hip “lowbrow” genres, like blues, rock, or drum and bass). Omnivorous consumption is “I like everything but country” or “I like everything but hip-hop.”
The Jack format combined that idea with the perceived influence of emerging media-player software (iTunes and the like) on listening practices. As R. Colin Tait writes, “When the radio industry launched the Jack FM format in 2000, it was based on the rise of download culture. MP3 listeners had become accustomed to listening to their favorite songs randomly and did not seem to mind when their favorite country song played after a classic metal tune.” People attributed a similar effect to the iPod, as in this Macworld opinion piece: “It brought the idea of ‘shuffle’ to listeners. With the iPod, and iTunes, you can listen to music at random. Instead of making choices, you can let fate choose what you listen to” (emphasis added). As individual listeners turned over control of their private listening to the arbitrary shuffle algorithm, radio stations programmed genre-hopping formats that mimicked the shuffle’s apparent randomness.
As individual listeners turned over control to the arbitrary shuffle algorithm, radio stations programmed genre-hopping formats that mimicked the shuffle’s apparent randomness
These days, streaming services from Apple Music to Spotify to YouTube don’t let fate choose what you listen to: They delegate the important task of making choices to carefully designed algorithms, as these articles detail. This approach builds on the Jack FM-style reshuffling of format and its relation to genre, reorienting both categories away from representing what Weisbard calls “publics” and to a representation of a mathematical relationship among numbers.
Before, demographics — populations defined through statistical or group-based social identities, like gender, race, or class — affected how both format and genre boundaries were drawn. As Weisbard explains, the “format system … rooted in divisions of age, gender, race, region, and economics” was more an attempt at the “formatting of publics” than it was an attempt to represent musical genres. According to Weisbard, formats and genres interact, but they’re fundamentally different: Formats categorize people; genres categorize music. However, the relationship between demographics and aesthetics is like a feedback loop: Aesthetic values incorporate and appeal to identity-based stereotypes and hierarchies, and demographic categories and demographic thinking affect the aesthetic criteria used to determine and differentiate genres. The concept of genre in pop music is in no way reducible to demographics — it functions in lots of ways — but demographically based social identities have significantly permeated our understanding of genre.
There are plenty of gender-based genres: “cock rock” and “dad rock” vs. the heavily feminized Top 40 pop. And American audiences parsed genre categories along racially demographic lines throughout the 20th century. Up through the middle of the century, the music industry used the demographic category of “race” — a synecdoche for “black” — as a genre category. Music by black blues and jazz artists was called “race music,” and record stores were racially segregated just like bathrooms and water fountains were, with black consumers shunted to a separate and unequal space (the “race records” section of the store).
The associations of races with genres have had lasting effects. For example, though country music grew out of both black and white folk traditions, the controversy around Beyoncé’s 2016 single “Daddy Lessons” shows that many industry professionals and fans consider country music a white genre. Even though the song is country enough to have earned Beyoncé an invitation to perform it with the Dixie Chicks at the 2016 Country Music Association Awards, the Grammy committee rejected it for consideration as a country song, and it was widely criticized by country music insiders as not country enough, as this Vanity Fair piece details.
Two of Beyoncé’s key influences, Michael and Janet Jackson, used the associations between genre and racial demographics to craft genre-blending works depicting cross-racial cooperation, as Tamara Roberts argues in “Michael Jackson’s Kingdom: Music, Race, and the Sound of the Mainstream”: “Beat It” mixes R&B with Eddie Van Halen’s metal guitar, and the tracks on Rhythm Nation 1814 survey hip hop, hard rock, and pop to depict the multiculturally harmonious polity named by the album title. The Jacksons used the feedback between demographic identity categories and musical genre to make artistic statements about antiracism and multiculturalism.
But now that the industry has moved away from demographics and toward algorithmic format generation, it has become harder to use genre to make a political statement as the Jacksons did. Whereas demographics track membership in a politically-defined group, the new formats are based on categories that track (often individual) psychological characteristics. In other words, they have become psychographic.
If demographics conceives of populations in political terms (that demo- in demographics resonates with the demos of democracy), psychographics, which emerged as a field of market research in the mid-1960s, originally conceived of populations in psychological terms. Psychography forefather Emanuel Demby defined the field in the Spring 1994 issue of Market Research as “the use of psychological, sociological, and anthropological factors, such as benefits desired (from the behavior being studied), self-concept, and lifestyle (or serving style) to determine how the market is segmented” or, more simply, “the psychological profile of a population.”
Replacing essentializing stereotypes with abstractions that better account for intersectionality, psychometrics superficially resembles identity-based political categories
Demographics divide populations by conceptual structures that treat perceivable outward appearance (like phenotype, secondary sex characteristics, vocal timbre, or accent) as representations of inner character and capacity. Psychographics cut out the middleman of social identity and purport to study inner character and capacity themselves, “cluster[ing] people by their tendency to think or act in a certain way,” according to Demby, and not in terms of a identifiable proxy.
Though psychographic profiling has been around for decades, big data analytics and distributed computing have made it easier and more powerful to execute and implement, capable of targeting not just populations or market segments, but individuals. This ever more fine grained abstraction can break down blunt identity categories into their “intersectional” segments and supposedly predict future behavior based not on a person’s demographic group membership, but their actual past behavior. Such big-data driven psychographics (often called “psychometrics”) purport to be capable of both perceiving and predicting individual preferences and behaviors. Replacing essentializing stereotypes with abstractions that better account for intersectionality and individual situatedness, psychometrics represents identity-based political categories in a way that superficially resembles the way progressive academics have urged for decades.
Alexander Nix, the CEO of the now notorious psychometrics firm Cambridge Analytica, can come across like a gender studies major (though without the associated structural critique). He was quoted in a report published in the Swiss paper Das Magazin saying that it’s “ridiculous” to think that, for example, “all women should receive the same message because of their gender — or all African Americans because of their race.” The report details how the firm claimed the capability of “measuring people’s personality from their digital footprints” to target voters in the U.S. (for the Trump campaign) and the UK (for the pro-leave Brexiters). Regardless of the actual effectiveness of Cambridge Analytica’s analysis (which is widely disputed), the way they describe the problems with conventional demographic categories clearly illustrates the difference between psychometrics and demographics.
The rise of psychometric analysis has changed the way we think and talk about genre and format in pop music. As companies like YouTube begin to use psychometric techniques, using people’s online behaviors to define genre tags, the distinction Weisbard draws between formats (industry-driven categorizations of people) and genre (scene-driven categorizations of aesthetics) starts to break down. This breakdown is symptomatic of broad transformations in how we understand relations among sounds and people. Psychometrics convert inherently qualitative phenomena like moods and preferences into a specific type of mathematical relationship.
The quintessential example of this kind of psychographic categorization was Beats Music’s “The Sentence” feature, which presented users with a Mad-Lib-like sentence to complete with drop-down menus to program a stream of music: “I’m [pick a place] & feel like [pick an activity] with [pick a group of people] to [pick a genre of music].” Options in the “& feel like” menu were mainly verbs that closely tracked affects, emotions, and moods (e.g., “crying” or “winning”).
When Beats became Apple Music, that slippage between activity and affect became more explicit: Apple Music lets users pick playlists by “activity or mood.” Here, attitudes and moods assume the role once played by formats like Quiet Storm, AOR, or Top 40 in traditional broadcast radio. And broadcast radio, in turn, is increasingly adopting classic psychographics as a way to reconceive format in more brand-like terms, “where the style, feel and attitude of your station — its ‘stationality’ — resonates with the personality and emotional needs of your core listeners,” in the words of the psychographic analysis firm Mark Kassof & Co.
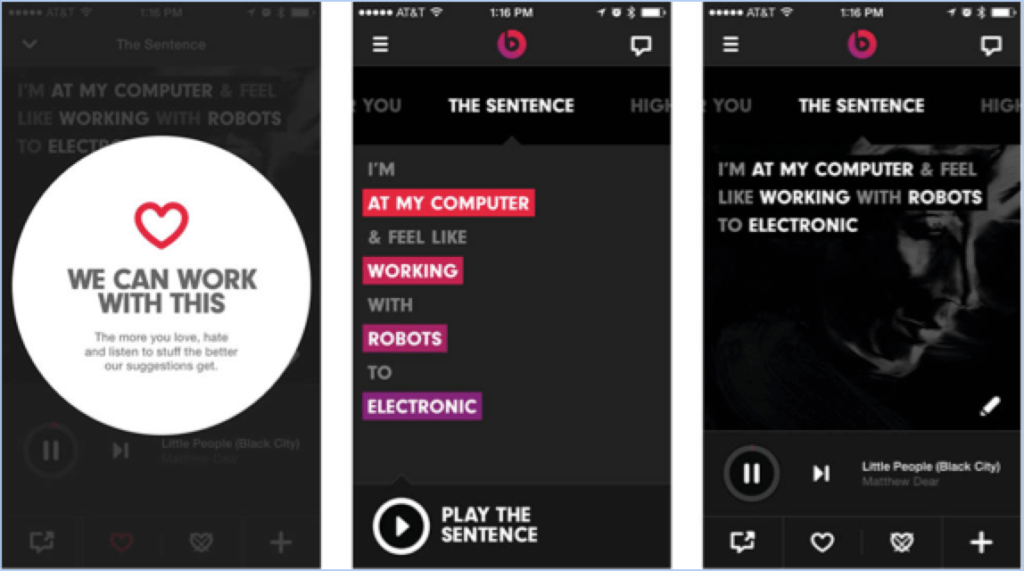
Psychometrics takes this collapse of format into genre even further, shifting not just the relationship between format and genre but the underlying concept of genre itself. Music scholars have conventionally understood genre as “a type of category that refers to a particular kind of music within a distinctive cultural web of production, circulation, and signification.” Psychometrics transforms the kind of web (of production, circulation, and signification) that genre spins. With platforms like Soundcloud encouraging artists to use as many hyperspecific genre and subgenre tags as possible, genre functions explicitly as metadata. Here, the web isn’t social or “cultural”; it’s mathematical. As philosopher Mary Beth Mader argues in Sleights of Reason, “the actual social relations between groups of people are masked in these figural expressions that employ the specific features of mathematical objects to characterize people and groups of people … endogenous mathematical traits that are superimposed on the social objects studied, rather than discovered in them.” In other words, when psychometrics substitutes mathematical relations (quantitative relations among numbers derived from metadata tags) for social and cultural relations, the aesthetic properties traditionally captured by genre (taste, status, value) become mathematical properties.
Psychographics cut out the middleman of social identity and purport to study inner character and capacity themselves, and not in terms of a identifiable proxy
This presents several problems. First, there’s conceptual accuracy: Aesthetic properties are inherently qualitative phenomena, and psychometrics redescribes them quantitatively. Psychometric aesthetics don’t describe relations among sounds but relations among numbers. Psychometric approaches to music reshape relations among sounds and the people who make and hear them so they embody a specific numerical relation: the normalized statistical distribution. This brings us to the second problem, which is political. Hegemonic institutions (the state, corporations) use statistical normalization along these lines to represent what a just and healthy society, market, and individual look like, to maintain longstanding relations of privilege and domination. Operating in this broader social context, psychometric approaches to music translate a politically unjust ideal into an equally suspect aesthetic ideal.
When music is distributed on platforms that allow and encourage tagging, genre and mood are treated as mathematical relationships among metadata. As researchers at Sun Labs and the University of Montreal explain, classifying music with tags reduces the qualitative features connoted and denoted by the words in the tag to one specific quantitative relationship: the frequency of a variable. For example, the Sun Labs study examined frequency of user-generated tags such as “rock” or “electronica,” “female vocals,” “love, favorite,” and “seen live” or “I own it” in a set of song metadata and compared this to the frequency with which those tags appeared when an algorithm generated tags for those same songs. The researchers describe their project as an attempt to “predict tags from acoustic features” of sound waves. Substituting the quantitative features of waveforms for the experienced aesthetic qualities of music, their method illustrates how psychometric methods reconfigure genre from an aesthetic to a mathematical phenomenon.
This has made it possible for the music industry to supplant the social relations structured though music with networked relations dictated by math. Some industry consultants encourage artists to use tags to create a mathematical relationship, to encourage audiences to see their work as linked to other, more famous artists despite having little in common with them geographically, socially, or culturally — the sorts of determinants that once placed artists (and fans) in a scene. For example, one website advises artists using Soundcloud and Bandcamp that “tagging is also the easiest way to force-associate your work with those who influence you, and communities to which you want to gain access.” The tag creates a mathematical relationship between the track, all other instances of that tag, and the tag’s networked associations. Another music-industry oriented blog instructs artists to use tags describing “main genre … mood, and location.” Creating a mathematical relation where no concrete social one (yet) exists helps artists promote their work and supposedly makes it easier to reach the ears of fans and A&R reps.
When streaming services use social tags to create individualized programming, they effect the same change to formats, shifting the kind of relationship it describes from a market or population segment to a networked distribution. Whereas traditional broadcast radio formats are demographic, YouTube’s streams are psychometric, created by tracking individual behavior, not by assuming population membership. Writing in The Outlook, Jeff Ihaza explains that YouTube’s system “uses implicit details about viewing habits. Data such as the amount of time spent watching a video and a video’s click-through rate are analyzed to create a sampling from which to serve recommendations.” He claims that “YouTube’s recommendations are so smart — and account for subtleties in ways that other platforms still don’t — that it effectively became a radio station for underground labels to scoop up fans.” Psychometric recommendation systems track the similarities among songs not in terms of their sound but as a function of the relative normalcy of listener/user behavior: Songs that elicit similar distributions of specific user behaviors it decides must be musically and aesthetically related.
As was the case with social tags, YouTube’s approach to format reduces qualitative phenomena like aesthetics and taste to a particular kind of mathematical relationship: rate and frequency of user behavior. As Kariann Goldschmitt and Nick Seaver argue in The Cambridge Companion to Digital Music and Culture, streaming services use differences in their recommendation systems to define their brands against the competition, effectively treating the algorithms behind their services the way broadcast radio stations treat format. Instead of anchoring differences in format in the perceived differences among audiences and population/market segments, streaming services anchor it in the proprietary features of the specific mathematical formula each service uses to calculate the similarities among song metadata.
Psychometric approaches to pop music don’t just affect how we classify it; they restructure the way we abstract from artworks and sensations to our ideas, thoughts, and concepts about art
Observed and analyzed psychometrically, then, formats and genres both describe the same thing: mathematical relationships. Perhaps this is why Ihaza is willing to collapse formats and genres into each other. In his article, he describes “lo-fi house” as a streaming format curated by YouTube that evolved into a genre as it was taken up by artists and listeners, with characteristic aesthetic features (hissing audio that recalls analog recordings, visual associations with 1980s club/rave fashion), labels, and artists.
Psychometric approaches to pop music don’t just affect how we classify it, though; they restructure the way we abstract from artworks and sensations to our ideas, thoughts, and concepts about art. They transform the kinds of abstractions we use to conceive and describe aesthetic phenomena like beauty, harmony, timbre, noise, and so on. Rather than use concepts to abstract aesthetic properties from sensory experiences — like Kant’s concepts of beauty and sublimity, for instance — they use mathematical ratios. When we use this abstraction to analyze and evaluate songs, aesthetics becomes just another type of statistical distribution.
This aligns the way we understand and appreciate music with the ways mathematical abstraction are used in other applications, like policing and finance, to intensify social inequity. If this method of abstraction is used broadly across our society in ways that systematically benefit historically advantaged groups, then it’s likely that our habituation to it will transfer over to how we think about, experience, and evaluate art.
Western music theory has always used math to temper the spectrum of audible frequencies into distinct, rationally related pitches. When we use statistical normalization to temper our hearing, we train ourselves to hear in precisely the terms that hegemonic institutions use to police relations of domination, such as white supremacy. In The Sonic Color Line, Jennifer Lynn Stoever calls “listening’s epistemological function as a modality of racial discernment” the “listening ear.” The listening ear works by “suppress[ing] and reduc[ing] an individual’s myriad, fine-grained embodied listening experiences by shunting them into narrow, conditioned, and ‘correct’ responses that are politically, culturally, economically, legally, and socially advantageous to whites.”
Because they are calibrated to a society that is already racist, music psychometrics train our listening ears to hear – in music and in general – the same mathematical relationships that treat white supremacy’s racially disproportionate distribution of risk and reward, life and death, as normal, rational, and pleasurable. When we put this listening ear to use, we reproduce those racially disproportionate distributions and make them even more “normal” in the statistical sense. Psychometric approaches to music affect much more than the relation between format and genre. Because they temper our listening ears, they have a direct impact on how we perceive and act in the world.