Amid accelerating environmental breakdown, widespread socioeconomic instability, and emergent forms of fascism, if there’s one consistency, it’s the sense of a complete lack of consistency: They all could be linked to a supposed “trust deficit” that a range of researchers, journalists and organizations have argued is currently worsening, what sociologist Gil Eyal has described as a “crisis of expertise.” On this view, more and more of the public regards the authority of both formal experts and institutions with suspicion, refusing to “believe the science” or the supposedly good intentions of those entities. This suspicion is bound up with a deeper sense of instability and anxiety, as both a contributing cause and a mounting effect.
Feeding both the mistrust and the anxiety is the idea of accelerating technological change, with artificial intelligence and machine learning being particularly concerning. Many fear, for instance, that algorithms will eliminate jobs through automation and exacerbate existing forms of injustice. And these fears are well-founded: Specific uses of these technologies — from crime prediction to employment verification to controlling the issuing of medications — and their associated modes of thought (i.e. a purely quantifiable approach to human existence and relations) will at best destabilize and at worse further dehumanize people, just as previous cycles of industrial and societal automation have done. While AI developers and their boosters may insist that “this time, it’s different,” it’s not clear what, if anything, has changed: As Judy Wajcman has argued, those making such promises often have little to say about what is different about their approach, and even less when those promises are broken.
This agency’s work on trust will be the work on trust in the U.S. as far as these technologies — for crime prediction, employment verification, controlling the issuing of medications — are concerned
It would seem like good news, then, that there is a plan to try to soothe these anxieties so that people can feel as though they can work with AI technologies rather than against them: a plan to develop trust in AI. But if, in such planning, the idea of trust itself is misconceived, this becomes more bad news. Such is the case with this U.S. Executive Order — signed by Donald Trump and carried over to the Biden administration — that is meant to ensure “reliable, robust, and trustworthy systems that use AI technologies.” It designates the National Institute of Standards and Technology (NIST) — a branch of the Department of Commerce that establishes measurement standards — as the lead organization in this project, which is tasked with developing rubrics for what such “trustworthy systems” might look like and how they might be assayed.
Its work on trust will therefore be, to a certain degree, the work on trust in the U.S. as far as these technologies are concerned: It will directly inform public standards around AI and how AI systems are subsequently developed. The U.S. government’s status as a primary purchaser of new technologies, combined with NIST’s role in setting technical expectations for said government — around system security, biometric data sharing, and a host of other areas — means that NIST guidance, however non-binding it might be in theory, often serves as the rules of the game in practice. The “secure hash algorithms” developed by NIST are an illustrative example: These have been adopted so broadly that they serve as the linchpin of what “secure” internet browsing means today. Its standards for what make an AI system “trustworthy” could have a similar normalizing effect.
The first fruits of NIST’s “trust” project, a recently released paper called “Trust and Artificial Intelligence,” focuses on how the institution defines “trust in AI” and how that trust might be modeled. Its definition, however, makes plain its priorities. Rather than define trustworthy systems with respect to their consequences or differential impacts, NIST focuses strictly on how they are perceived by “users,” who are defined in a restrictive and reductive way. Moreover, in assessing whether AI systems are trusted by these users, it does not bother to ask whether the systems themselves are even worth trusting.
Any kind of infrastructure, including algorithmic systems, have impacts that are not evenly distributed. As algorithms are introduced for everything from facial recognition to hiring to recidivism prediction, they are invariably met with valid concerns about the effects they will have on already marginalized communities. The history of concern, complaint, and critique about these algorithmic systems is as long as the history of the systems themselves.
So it is disconcerting that the NIST report, in a section called “Trust Is a Human Trait,” does not acknowledge or cite from that history of concern but instead quotes approvingly from a evolutionary psychology paper that basically argues that we are destined by natural selection to be bigots.
This sets the stage for how NIST will go on to conceptualize “trust”: not as a complex, historically conditioned social phenomenon that takes many different forms in different and often conflicting contexts but as something very simple. So simple, in fact, that it can be resolved into an equation:

For this model to make sense, you need — among other things — for trust to be a matter of two fixed, contextless parties and their more or less isolated encounters with each other. Interactions with AI, that is, must live in a little two-party bubble, just as it does in this figure from the report:
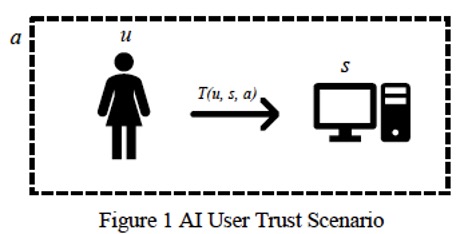
After ruling out any situation that doesn’t involve a single person electing to use a single AI system, the equation then defines trust as calculable in that scenario in terms of an array of the AI system’s design attributes and an array of user attributes. To evaluate how much the user trusts the system, you take the system’s security, resiliency, objectivity, and so on — all quantifiable attributes — and match them with, well, such attributes as the user’s gender, age, and personality type, which are also conceived as fully quantifiable and capable of being plugged into the equation above. In other words, NIST’s first step in handling people’s unease with systems that reduce the world to what is quantifiable is to reduce people’s unease itself to something quantifiable.
Tellingly, the paper illustrates a “user” with two hypothetical examples, which could be summed as: middle-aged lady who doesn’t use computers;
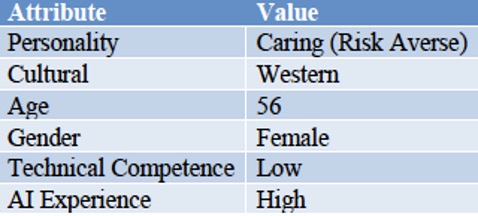
and the hip young programmer bro.
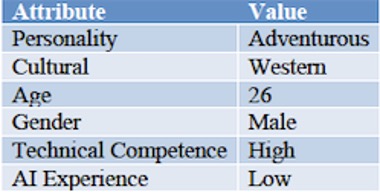
Their attributes, after being converted to quantities, are then evaluated with respect to the attributes of a particular AI system to determine the likelihood that the user will “trust” that system — understood here as their willingness to continue using it. So while the second user above might be young enough and male enough to have a smooth and trusting relationship with AI, the first user might be seen as lacking the necessary attributes.
In this setup, mistrust is not conceived as a matter of shortcomings or inconsistencies in algorithms’ broader designs or their impacts themselves but as a matter of a user perceptions. To fix “trust issues,” the users’ perceptions would need to change; changes to the algorithm are necessary only to alter those perceptions
None of this is how AI works in practice. In NIST’s model, as the diagram helpfully captures, there are two parties to an algorithmic interaction. In reality, there are many, many more. Algorithms are embedded in broader infrastructures of data collection, processing, refining and deployment, and as Daniel Neyland has documented, each of these stages involves many actors (programmers, marketers, corporate executives, and, infrequently, regulators) with many different concerns that end up reflected in the resulting software. What an algorithmic system that, say, matches users to possible purchases “does,” after all, is not simply match users to “all possible purchases” but those use cases that the system’s marketers have chosen to emphasize (what products have the highest profit margin?), what that system’s programmers had the time and budget to implement (what products were fastest to categorize and import?), and what sorts of user experience its researchers saw as worth testing.
But more pressingly, even if you consider a particular algorithm only in the context of its deployment, there are still more parties involved: One must consider the algorithm’s developers and deployers and their interests in a particular interaction. Can the user trust them? For example, the purpose of Amazon’s Alexa, we are told on its app store page, is to help users “listen to music, create shopping lists, get news updates, and much more.” But it is also to passively collect a user’s data, shipping it back to Amazon, who can then use it to produce anything from personalized advertisements to a reconstruction of users’ identity to enable further data mining.
Defining “trust” as the degree to which a user believes the algorithm can do its job evades the question of whether the user wants it to
As Nanna Bonde Thylstrup insightfully observes, the “big data hype” is fundamentally based on “the waste-related epiphany that seemingly useless data can be extracted, recycled, and resold for large amounts of money.” Reuse and dual-use, in other words, are part and parcel of how even AI developers see their work. Thus algorithmic interactions are not, as NIST portrays, about a single user trusting a single algorithm for a single purpose, but instead they are multifarious exchanges for multifarious purposes among multiple parties, some of which are obscured from any individual user.
Examples like Alexa, however, are not fully representative, because they represent situations where — to some degree, at least — the user can be said to have opted into a service or an interaction with an algorithm. But in more and more cases, users have algorithmic systems imposed on them, as with, for instance, work-tracking software that allows employers to fire people for not keeping pace. (This is in line with the long history of workplace automation, which is often deployed specifically to eliminate jobs, as detailed in this paper.) In such situations, defining trust as the degree to which a user believes the algorithm can do its job completely evades the question of whether the user (let alone society at large) even wants it to. That a particular user “uses” an algorithm is hardly proof that they trust it or its logic or the other actors behind it. Rather, it may reflect the fact that they don’t have a choice. They may not be not trusting but trapped.
Users are often fully aware of the sometimes treacherous nature of algorithmic systems and the multiple motivations of the organizations behind them but feel they have no alternative but to use them. When Cami Rincón, Corinne Cath-Speth, and I interviewed trans people about their concerns with voice-activated AI like Alexa and Siri for this paper, they weren’t focused on usability (e.g. “can I trust Siri to provide an answer to a question”); they were focused on the agendas of Apple and Amazon. That is, they “trusted” that the algorithms could do what they were expected to do but not that what they were doing wasn’t ultimately very, very bad for the user, extracting their personal information and desires in order to generate ever more precise efforts to extract their money as well. In other words, these systems are untrustworthy because they work. It’s precisely because they meet the ideals of reliability and resiliency that NIST highlights as components of trust that certain groups of users find them harmful and unacceptable.
This highlights the harm that NIST’s definition of trust allows for and in some ways enables. If trust is modeled as strictly between the user and the algorithm, oriented around a single, discrete task, then user distrust of companies like Apple and Amazon can be dismissed as irrelevant, either because the users’ reliance on the system is taken as sign of trust or because qualms about the algorithm’s developers or their other potential purposes is outside the definition’s scope. The definition further implies that “trust” requires users themselves be categorizable and categorized in ways that fit simplistic and hegemonic ideas of humanity.
Developers can brag about how “trusted” their algorithms are by regulators — and trusted by the regulators’ own standards, to boot! — while the systems’ parallel impacts or indirect harms and those experiencing them are ignored.
This inadequate definition of trust isn’t just NIST’s problem or failure. The idea of algorithmic trust as focused on the user and algorithm alone is prevalent in a lot of the work in the fields of computer science and human-computer interaction. In a meta-analysis that looked at 65 studies on the topic of trust, the closest the authors came to identifying broader structural and organizational concerns as a factor was in their discussion of “reputation” — specifically, the algorithm’s reputation, not the wider agenda of developers.
In some respects, NIST’s limited view is not surprising: The researchers’ task for this report was to explicitly model trust, and since broader contexts are hard to model, they have simply been sidelined from the start. This is precisely the problem. It’s not just that NIST gets trust wrong; it’s that it can’t possibly get it right if trust is treated as merely a technical problem. Trust isn’t not technical, but it isn’t just technical, either. It is ultimately political. Giving the task of defining trust to a technical standards organization is ultimately a way of eliding those political ramifications. Trust is not just about how reliably an algorithm does its work but what that work is, who that work is for, and what responsibilities come with its development and deployment. NIST is not equipped to answer these questions. It is capable only of burying them.
Rather than models aimed at instrumentalizing trust, what we desperately need is to take a step back and confront algorithmic systems as a political phenomenon: as systems that demand public and democratic accountability. This is not an easy task: It raises questions not just about the design of algorithmic systems but about the structure of work, the vast ecosystem of relations between companies, and what futures we collectively want to build toward. But these concerns do not go away if we mask them with a technocratic view of algorithmic systems and their regulation. They simply lurk and rot, returning to haunt us in a more malevolent form. We may find ourselves with AI systems that have perfect scores on measures of algorithmic trust, even as the world in which they operate has become more distrustful and destabilized than ever.